Artificial intelligence predicts research trends in quantum physics
15.01.2020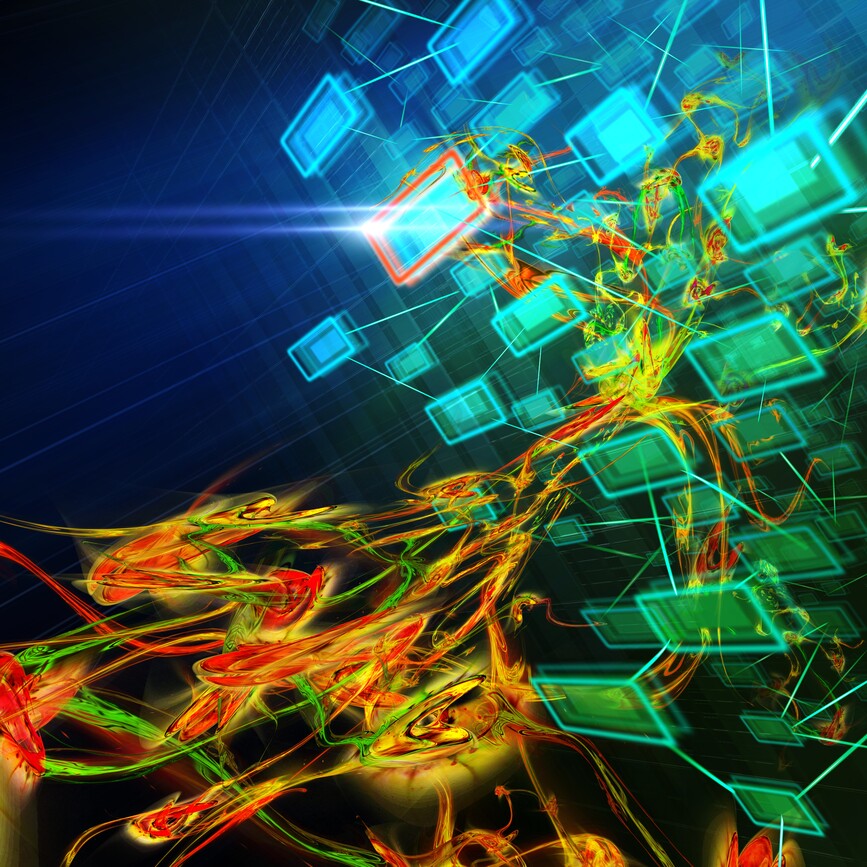
The increasing specialization of science sometimes makes it difficult for researchers to develop new projects that go beyond their own area of expertise. "Around 50 articles appear every day in quantum physics alone, and this is only one subfield of physics. You have to make an effort just to be able to take in all the titles", says Mario Krenn from the University of Toronto.
Together with Anton Zeilinger, quantum physicist at IQOQI-Vienna (Austrian Academy of Sciences) and the University of Vienna, Krenn has now demonstrated an innovative solution to this problem in the journal "Proceedings of the National Academy of Sciences" (PNAS): a computer-aided system for efficient processing of quantum physics knowledge. "First, we automatically created a list of around 6,500 key concepts in quantum physics from books, specialist articles and Wikipedia. Then we defined these terms as nodes in a network. Two concepts are combined if they have been mentioned concurrently in the specialist literature. The more often this happens, the stronger the connection", Krenn explains.
"The approach has the potential to significantly change the way science is done", says Anton Zeilinger. The system can in principle be used for any research area. "Before we generalize, we would like to work on better interpreting the results of the quantum physics network. There are already several scientists around the world who are interested in working with us on this", Krenn adds.
750,000 research articles analyzed
In this way, the researchers created a so-called semantic network, which shows the connections between different concepts of quantum physics. To investigate the connections, Krenn and Zeilinger analyzed the abstracts of 750,000 research articles from quantum physics published since 1919. "The network is a simple representation of knowledge, but it has accurately reflected past developments in quantum physics", Krenn says.
As the next step, the two researchers used this network on the one hand and artificial intelligence on the other to predict future developments in quantum physics research. "We input the semantic network into a neural network. This learned to use the data from 2010 to predict the state of the semantic network in 2015 to a good approximation. The neural network was able to estimate with high probability which concepts not yet connected in 2010 would be jointly researched by 2015", Krenn explains.
The artificial intelligence trained in this way can thus be used to create a forecast based on the latest semantic network as to which concepts will soon be researched together.
Suggestions for future research
"Currently, only about five percent of the concepts are linked in the network. Our system can, however, make proposals for researchers to search for new, unexpected subject areas", the quantum physicist continues. Krenn describes the predictions of the neural network as only part of the forecasts. "We use tools from network theory to search for unusual connections, for instance two core concepts that are strongly predicted but significantly differ in their meaning", Krenn says. Such interdisciplinary connections sometimes seem very surprising and unorthodox. Therefore, the researchers also developed a method that can be used to generate promising research proposals that overlap with a specific field.
Publication:
"Predicting Research Trends with Semantic and Neural Networks with an application in Quantum Physics", Mario Krenn, Anton Zeilinger, PNAS 2019 (Open Access)
DOI: https://doi.org/10.1073/pnas.1914370116